Integrating Generative AI with Knowledge Graphs for Enhanced Data Analytics
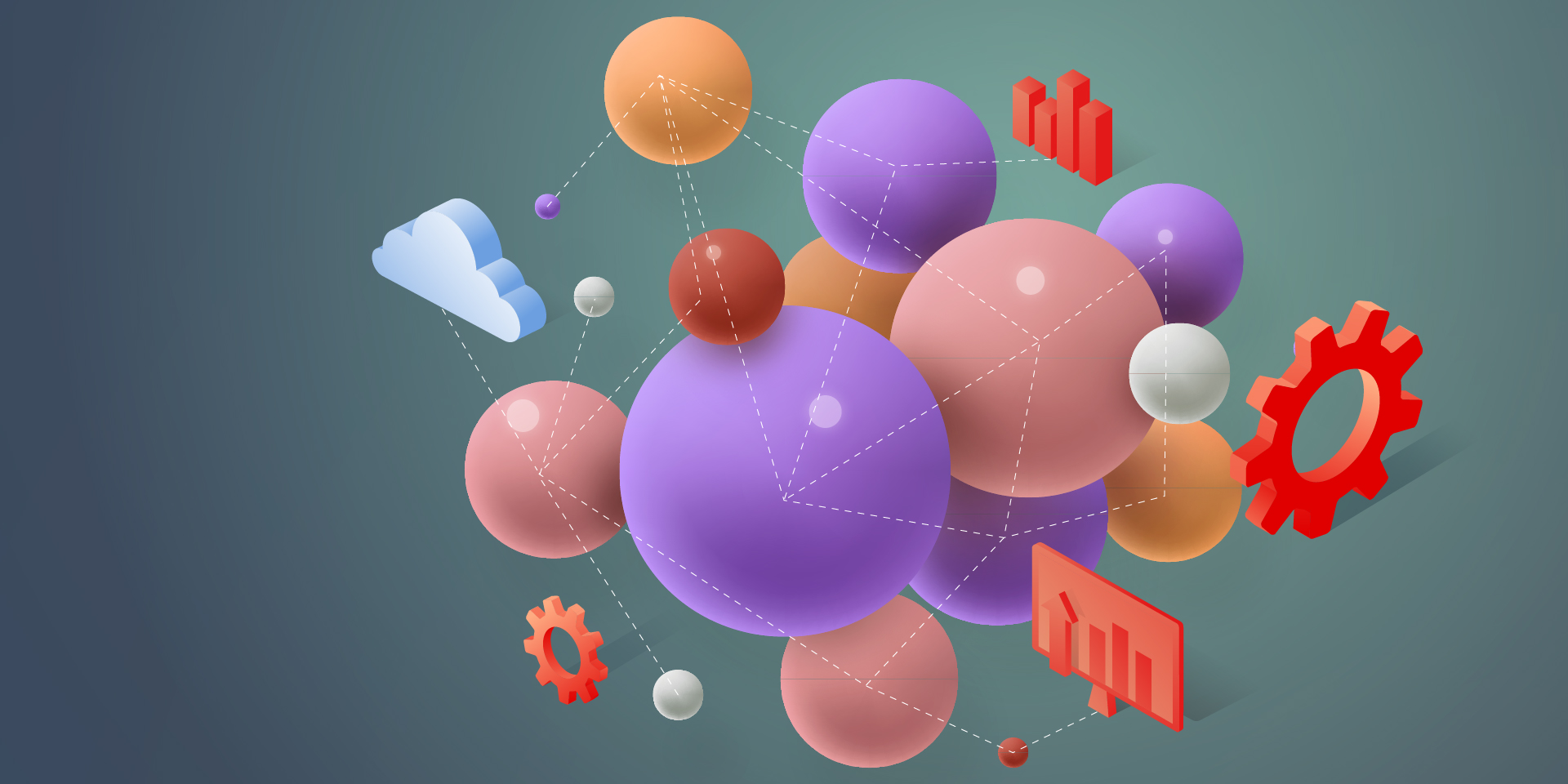
The integration of generative AI into data analytics is transforming business data management and interpretation, opening vast possibilities across industries.
Recent statistics from a Gartner survey indicate significant strides in the adoption of generative AI: 45% of organizations are now piloting generative AI projects, with 10% having fully integrated these systems into their operations. This marks a considerable increase from earlier figures, demonstrating a rapid adoption curve. Additionally, by 2026, it’s predicted that more than 80% of organizations will use generative AI applications, up from less than 5% just three years prior.
Combining Generative AI and Knowledge Graphs for Data Analytics
The potential impact of combining generative AI with knowledge graphs is particularly promising. This synergy enhances data analytics by improving accuracy, speeding up data processing, and enabling deeper insights into complex datasets. As adoption continues to expand, the mentioned technologies will transform how organizations leverage data for strategic advantage.
This article details the specific benefits of generative AI and knowledge graphs and how their integration can boost data-based decision-making processes.
Maximizing Generative AI Potential in Data Analytics
Generative AI has revolutionized data analytics by automating tasks that traditionally required significant human effort and by providing new methods to manage and interpret large datasets. Here is a more detailed explanation of how GenAI operates in various aspects of data analytics.
Rapid Summarization of Information
GenAI’s ability to swiftly process and summarize large volumes of data is a boon in situations that demand quick insights from extensive datasets. This is especially critical in areas like financial analysis or market trend monitoring, where rapid information condensation can significantly expedite decision-making processes.
Enhanced Data Enrichment
In the initial stages of data analytics, raw data is often unstructured and may contain errors or gaps. GenAI plays a crucial role in enriching this raw data before it can be effectively visualized or analyzed. This includes cleaning the data, filling in missing values, generating new features, and integrating external data sources to add depth and context. Such capabilities are particularly beneficial in scenarios like predictive modeling for customer behavior, where historical data may not fully capture current trends.
Automation of Repetitive Data Preparation Tasks
Data preparation is often the most time-consuming part of data analytics. GenAI helps automate these processes with unmatched precision and speed. This not only enhances the efficiency and accuracy of data preparation but also improves data quality by quickly identifying and correcting inconsistencies.
Complex Data Simplification
GenAI expertly simplifies complex data patterns, making them easy to understand and accessible. This allows users with varying levels of expertise to derive actionable insights and make informed decisions effortlessly.
Interactive Data Exploration via Conversational Interfaces
GenAI uses Natural Language Processing (NLP) to facilitate interactions, allowing users to query data in everyday language. This significantly lowers the barrier to data exploration, making analytics tools more user-friendly and extending their use across different organizational departments.
The Use of Knowledge Graphs in Data Analytics
Knowledge graphs prove increasingly useful in data analytics, providing a solid framework to improve decision-making in various industries. These graphs represent data as interconnected networks of entities linked by relationships, enabling intuitive and sophisticated analysis of complex datasets.
What Are Associative Knowledge Graphs?
Associative knowledge graphs are a specialized subset of knowledge graphs that excel in identifying and leveraging intricate and often subtle associations among data elements. These associations include not only direct links but also indirect and inferred relationships that are crucial for deep data analysis, AI modeling, and complex decision-making processes where understanding subtle connections can be crucial.
Associative Knowledge Graphs Functionalities
Associative knowledge graphs are useful in dynamic environments where data constantly evolves. They can incorporate incremental updates without major structural changes, allowing them to quickly adapt and maintain accuracy without extensive modifications. This is particularly beneficial in scenarios where knowledge graphs need to be updated frequently with new information without retraining or restructuring the entire graph.
Designed to handle complex queries involving multiple entities and relationships, these graphs offer advanced capabilities beyond traditional relational databases. This is due to their ability to represent data in a graph structure that reflects the real-world interconnections between different pieces of information. Whether the data comes from structured databases, semi-structured documents, or unstructured sources like texts and multimedia, associative knowledge graphs can amalgamate these different data types into a unified model.
Additionally, associative knowledge graphs generate deeper insights in data analytics through cognitive and associative linking. They connect disparate data points by mimicking human cognitive processes, revealing patterns important for strategic decision-making.
Generative AI and Associative Knowledge Graphs: Synergy for Analytics
The integration of Generative AI with associative knowledge graphs enhances data processing and analysis in three key ways: speed, quality of insights, and deeper understanding of complex relationships.
Speed: GenAI automates conventional data management tasks, significantly reducing the time required for data cleansing, validation, and enrichment. This helps decrease manual efforts and speed up data handling. Combining it with associative knowledge graphs simplifies data integration and enables faster querying and manipulation of complex datasets, enhancing operational efficiency.
Quality of Insights: GenAI and associative knowledge graphs work together to generate high-quality insights. GenAI quickly processes large datasets to deliver timely and relevant information. Knowledge graphs enhance these outputs by providing semantic and contextual depth, where precise insights are vital.
Deeper Understanding of Complex Relationships: By illustrating intricate data relationships, knowledge graphs reveal hidden patterns and correlations which leads to more comprehensive and actionable insights that can improve data utilization in complex scenarios.
Example applications
Healthcare:
- Patient Risk Prediction: GenAI and associative knowledge graphs can be used to predict patient risks and health outcomes by analyzing and interpreting comprehensive data, including historical records, real-time health monitoring from IoT devices, and social determinants of health. This integration enables creation of personalized treatment plans and preventive care strategies.
- Operational Efficiency Optimization: These technologies optimize resource allocation, staff scheduling, and patient flow by integrating data from various hospital systems (electronic health records, staffing schedules, patient admissions). This results in more efficient resource utilization, reduced waiting times and improved overall care delivery.
Insurance, Banking & Finance:
- Risk Assessment / Credit Scoring: Using a broad array of data points such as historical financial data, social media activity, and IoT device data, GenAI and knowledge graphs can help generate accurate risk assessments and credit scores. This comprehensive analysis uncovers complex relationships and patterns, enhancing the understanding of risk profiles.
- Customer Lifetime Value Prediction: These technologies are utilized to analyze transaction and interaction data to predict future banking behaviors and assess customer profitability. By tracking customer behaviors, preferences, and historical interactions, they allow for the development of personalized marketing campaigns and loyalty programs, boosting customer retention and profitability.
Retail:
- Inventory Management: Customers can also use GenAI and associative knowledge graphs to optimize inventory management and prevent overstock and stockouts. Integrating supply chain data, sales trends, and consumer demand signals ensures balanced inventory aligned with market needs, improving operational efficiency and customer satisfaction.
- Sales & Price Forecasting: Otherwise, you can forecast future sales and price trends by analyzing historical sales data, economic indicators, and consumer behavior patterns. By combining various data sources, you get a comprehensive understanding of sales dynamics and price fluctuations, aiding in strategic planning and decision-making.
gIQ – data analytics platform powered by generative AI and associative knowledge graphs The gIQ data analytics platform demonstrates one example of integrating generative AI with knowledge graphs. Developed by Grape Up founders, this solution represents a cutting-edge approach, allowing for transformation of raw data into applicable knowledge. This integration allows gIQ to swiftly detect patterns and establish connections, delivering critical insights while bypassing the intensive computational requirements of conventional machine learning techniques. Consequently, users can navigate complex data environments easily, paving the way for informed decision-making and strategic planning. |
Conclusion
The combination of generative AI and knowledge graphs is transforming data analytics by allowing organizations to analyze data more quickly, accurately, and insightfully. The increasing use of these technologies indicates that they are widely recognized for their ability to improve decision-making and operational efficiency in a variety of industries.
Looking forward, it’s highly likely that the ongoing development and improvement of these technologies will unlock more advanced and sophisticated applications. This will drive innovation and give organizations a strategic advantage. Embracing these advancements isn’t just beneficial, it’s essential for companies that want to remain competitive in an increasingly data-driven world.
Check related articles
Read our blog and stay informed about the industry's latest trends and solutions.
see all articles