Generative AI in Automotive: How Industry Leaders Drive Transformation
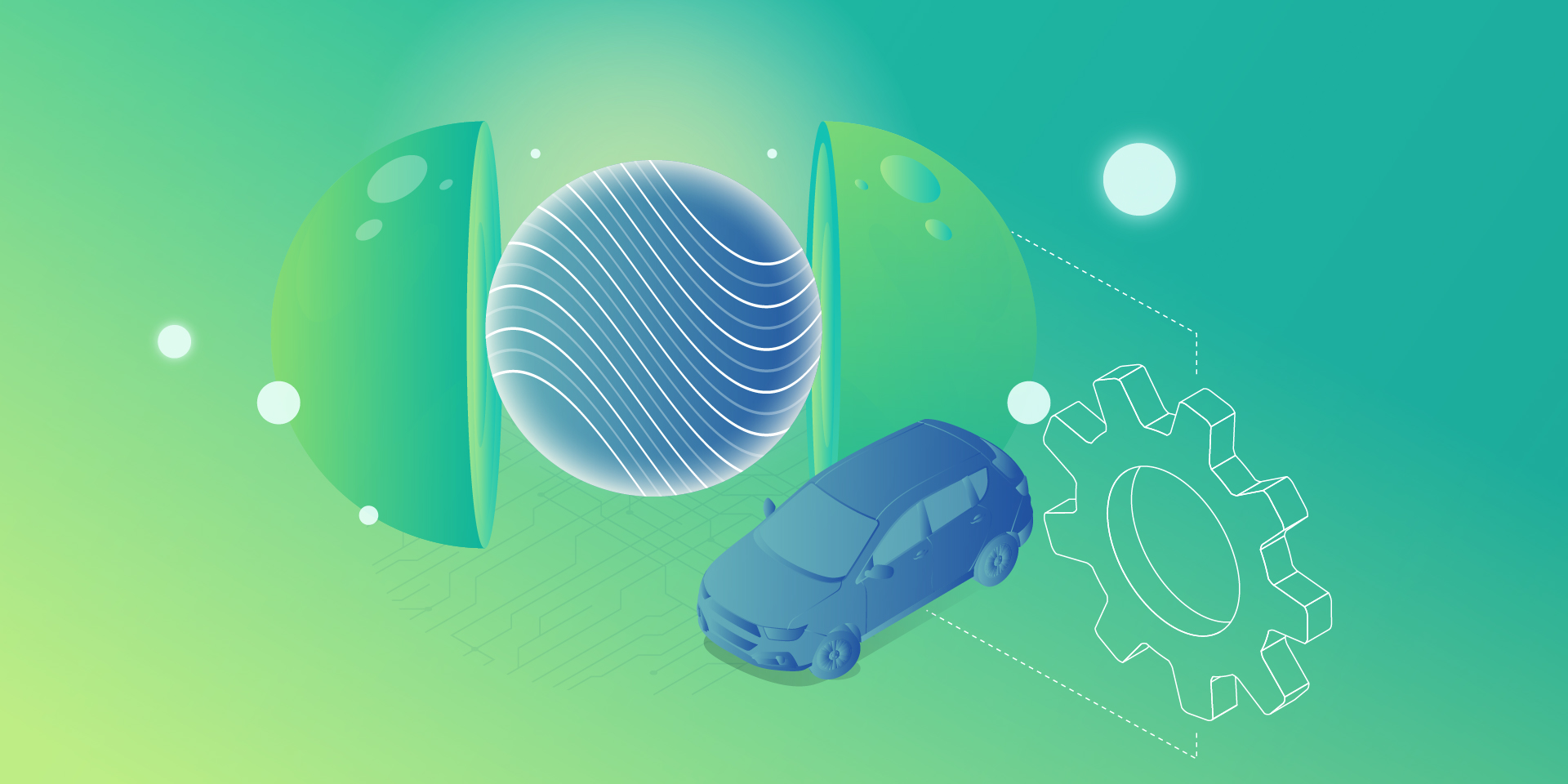
Generative AI is quickly emerging as one of the key drivers of automotive innovation. This shift is not just a future possibility; it’s already happening. In 2023, the generative AI market in the automotive sector was valued at approximately USD 387.54 million. Looking ahead, it’s projected to surge to about USD 2,691.92 million by 2032, demonstrating a robust Compound Annual Growth Rate (CAGR) of 24.03% from 2023 to 2032. Major Original Equipment Manufacturers (OEMs) are integrating sophisticated AI algorithms into various aspects of the industry, from vehicle design to enhancing customer interactions.
The impact of generative AI in the automotive sector is already evident. For instance, NVIDIA’s generative AI models empower designers to swiftly transform 2D sketches into intricate 3D models, significantly speeding up the design process and opening up new avenues for creativity and efficiency. Meanwhile, automotive manufacturing companies are exploring collaborations with tech giants to integrate advanced AI language models into their vehicles, enhancing the driving experience.
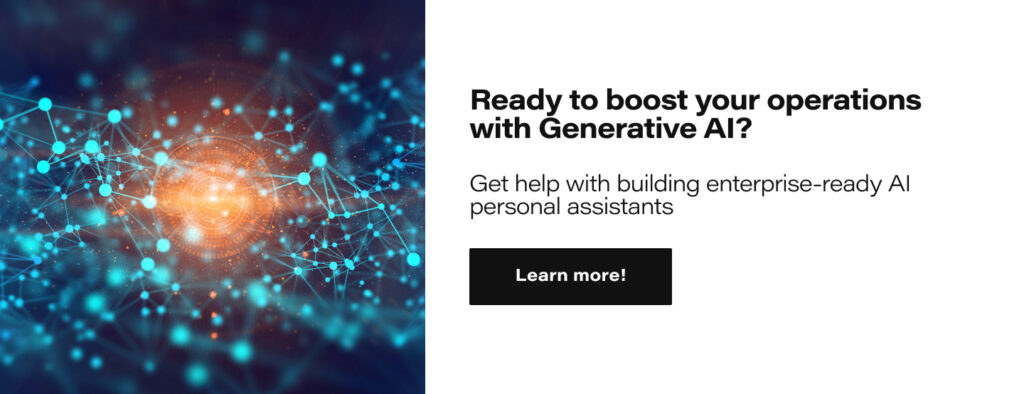
This article will explore how leading automotive players are leveraging generative AI to not only keep pace with the evolving demands of the market but also redefine mobility and automotive excellence.
Software-Defined Vehicles and Generative AI
The introduction of software-defined vehicles (SDVs) represents a significant shift in the automotive industry, moving beyond traditional performance metrics such as horsepower and chassis design to focus on software and digital capabilities. By 2025, it is estimated that vehicles could require as much as 650 million lines of code each. These vehicles heavily rely on software for critical operations such as driving assistance, navigation, and in-car entertainment systems.
The integration of generative AI in this domain further amplifies these capabilities. Generative AI is known for its ability to create and optimize designs and solutions, which is beneficial in improving both the software and hardware aspects of SDVs. It helps in generating efficient algorithms for vehicle control systems, contributes to the development of more effective and adaptive software solutions, and even assists in designing vehicle components for better performance and efficiency.
However, bringing generative AI into this landscape presents both unique opportunities and significant challenges.
The Opportunities and Challenges
- Challenges
Integrating advanced AI systems into modern vehicles is a complex and multifaceted task that demands technical expertise and careful attention to data security and privacy, particularly with the increasing reliance on data-driven functionalities in vehicles.
The automotive industry is facing a complex regulatory environment. With the growing importance of AI and data in-vehicle systems, it has become crucial to comply with various international regulations and standards, covering areas such as data protection, safety, and environmental impact.
One significant challenge for OEMs is the lack of standardization within the software-defined vehicles industry, which can complicate the development and integration of new technologies as there are no universal norms or protocols to guide these processes.
Internal transformation is also a critical aspect of this integration. OEMs may need to revamp their internal capabilities, processes, and technological infrastructure to use generative AI effectively.
- Opportunities
Integrating generative AI technology allows for more creative and efficient vehicle design, resulting in quicker prototypes and more innovative models.
It also allows for creating personalized vehicles that cater to individual user preferences like never before. In manufacturing, generative AI promotes more efficient and streamlined production processes, which optimizes resources and reduces waste.
Let’s explore how automotive manufacturers already use gen AI to boost their operations.
Generative AI Applications in the Automotive Industry
Generative AI’s integration into the automotive industry revolutionizes multiple facets of vehicle design, manufacturing, and user experience. Let’s explore these areas:
Design and Conceptualization
- Vehicle Design Enhancement: Artificial Intelligence is revolutionizing the vehicle design process by speeding up the initial phase of the design cycle. Generative design algorithms use parameters such as material properties, cost constraints, and performance requirements to generate optimal design solutions. For example, in vehicle body design, AI can propose multiple design options that optimize for aerodynamics and strength while minimizing weight. This enables quick visualization and modification of ideas.
Toyota Research Institute has introduced a generative AI technique to optimize the vehicle design process to produce more efficient and innovative vehicles. This approach allows designers to explore a wider range of design possibilities, including aerodynamic shapes and new material compositions.
- Digital Prototyping: The use of Generative AI technology makes it possible to create digital prototypes, which can be tested and refined extensively without the need for physical models. This approach is highly beneficial, as it enables designers to detect and correct potential design flaws early in the process.
BMW’s use of NVIDIA Omniverse is a significant step in design improvement. The company uses this platform to create digital twins of their manufacturing facilities, integrating generative AI to enhance production efficiency and design processes.
Manufacturing and Production
- Streamlining Manufacturing Processes: Generative AI significantly enhances the efficiency of manufacturing processes. Unlike traditional AI or machine learning models, generative AI goes beyond identifying inefficiencies; it actively generates novel manufacturing strategies and solutions. By inputting parameters such as production timelines, material constraints, and cost factors, generative AI algorithms can propose a range of optimized manufacturing workflows and processes.
BMW has implemented generative AI in a unique way to improve the scheduling of their manufacturing plant. In partnership with Zapata AI, BMW utilized a quantum-inspired generative model to optimize their plant scheduling, resulting in more efficient production. This process, known as Generator-Enhanced Optimization (GEO), has significantly improved BMW’s production planning, demonstrating the potential of generative AI in industrial applications.
- Supply Chain Resilience: In the context of supply chain management, particularly during challenges like the automotive microchip shortage, generative AI plays a crucial role. Unlike conventional AI, gen AI can do more than just analyze existing supply chain networks; it can creatively generate alternative supply chain models and strategies. The algorithms can propose diverse and robust supplier networks by leveraging data about supplier capabilities, logistics constraints, and market demands.
- Customized Production: With generative AI, it is now possible to create personalized vehicles on a large scale, meeting the growing demand for customization in the automotive industry.
Predictive Maintenance and Modelling
Traditionally, predictive maintenance relies on historical data to forecast equipment failures, but generative AI enhances this process by creating detailed, simulated data environments. This technology generates realistic yet hypothetical scenarios, encompassing a vast array of potential machine failures or system inefficiencies that might not be present in existing data sets.
The generative aspect of this AI technology is particularly valuable in situations where real-world failure data is limited or non-existent. By synthesizing new data points, generative AI models can extrapolate from known conditions to predict how machinery will behave under various untested scenarios.
In modeling, generative AI goes a step further. It not only predicts when and how equipment might fail but also suggests optimal maintenance schedules, anticipates the impact of different environmental conditions, and proposes design improvements.
Customer Experience and Marketing
One of the challenges in using generative AI, particularly in customer interaction, is the accuracy of AI-generated responses. An example of this was an error by ChatGPT, where it was tricked into suggestion of buying a Chevy for a dollar. This incident underlines the potential risks of misinformation in AI-driven communication, emphasizing the need for regular updates, accuracy checks, and human oversight in AI systems. Nevertheless, this technology offers many opportunities for improving the customer experience:
- Personalized User Experiences and Enhanced Interaction: AI’s capability to adapt to individual preferences not only enhances the driving experience but also improves the functionality of vehicle features.
For instance, in collaboration with Microsoft, General Motors is exploring the use of AI-powered virtual assistants that offer drivers a more interactive and informative experience. These assistants can potentially provide detailed insights into vehicle features and performance metrics and offer personalized recommendations based on driving patterns.
Also, Mercedes-Benz is exploring the integration of generative AI through voice-activated functionalities in collaboration with Microsoft. This includes leveraging the OpenAI Service plugin ecosystem, which could allow for a range of in-car services like restaurant reservations and movie ticket bookings through natural speech commands.
Example Applications
- Simplified Manuals: AI technology, enabled by natural language processing, has simplified the interaction between drivers and their vehicles. Beyond just responding to voice commands with pre-existing information, a generative AI system can even create personalized guides or tutorials based on the driver’s specific queries and past interactions.
Grape Up has developed an innovative voice-driven car manual that allows drivers to interact with their vehicle manual through voice commands, making it more accessible and user-friendly. With this technology, drivers no longer have to navigate through a traditional manual. Still, they can easily ask questions and receive instant verbal responses, streamlining the process of finding information about their vehicle.
- Roadside Assistance: In this scenario, generative AI can go beyond analyzing situations and suggesting solutions by creating new, context-specific guidance for unique problems. For instance, if a driver is stranded in a rare or complex situation, the AI could generate a step-by-step solution, drawing from a vast database of mechanical knowledge, previous incidents, and environmental factors.
- Map Generation: Here, generative AI can be used to not only update maps with real-time data but also to predict and visualize future road conditions or propose optimal routes that don’t yet exist. For example, it could generate a route that balances time, fuel efficiency, and scenic value based on the driver’s preferences and driving history.
- Marketing and Sales Innovation: Generative AI-enabled content engine is transforming the creation of digital advertising for the automotive industry. This content is tailored to meet the unique requirements of automotive brands and their consumers, thereby revolutionizing traditional marketing strategies.
Safety and Compliance
- Enhancing Vehicle Safety: Generative AI in vehicles goes beyond traditional AI systems by not only assisting drivers but also by creating predictive models that enhance safety features. It processes and interprets data from cameras and sensors to foresee potential road hazards, often employing advanced generative models that simulate and predict various driving scenarios.
- Regulatory Compliance: Similarly, gen AI helps automakers comply with safety standards and navigate complex regulation changes by monitoring performance data and comparing it against regulatory benchmarks. This allows automakers to stay ahead of the compliance curve and avoid potential legal and financial repercussions.
Autonomous Vehicle Development
- Simulation and Testing: Generative AI is crucial for developing autonomous vehicle systems. It generates realistic simulations, including edge-case scenarios, to test and improve vehicle safety and performance.
- Enhancing ADAS Capabilities: AI technology can improve essential Advanced Driver Assistance Systems (ADAS) features such as adaptive cruise control, lane departure warnings, and automatic emergency braking by analyzing data from various sensors and cameras. Generative AI’s strength in this context lies in its ability to not only process existing data but also to generate new data models, which can predict and simulate different driving scenarios. This leads to more advanced, reliable, and safer ADAS functionalities, significantly contributing to the evolution of autonomous and semi-autonomous driving technologies.
Conclusion
As the automotive industry accelerates towards a more AI-integrated future, the role of expert partners like Grape Up becomes increasingly crucial. Our expertise in navigating the intricacies of AI implementation can help automotive companies unlock the full potential of this technology. If you want to stay ahead in this dynamic landscape, now is the time to embrace the power of generative AI. For more information or to collaborate with Grape Up, contact our experts today.
Check related articles
Read our blog and stay informed about the industry's latest trends and solutions.
see all articles