How to Make Your Enterprise Data Ready for AI
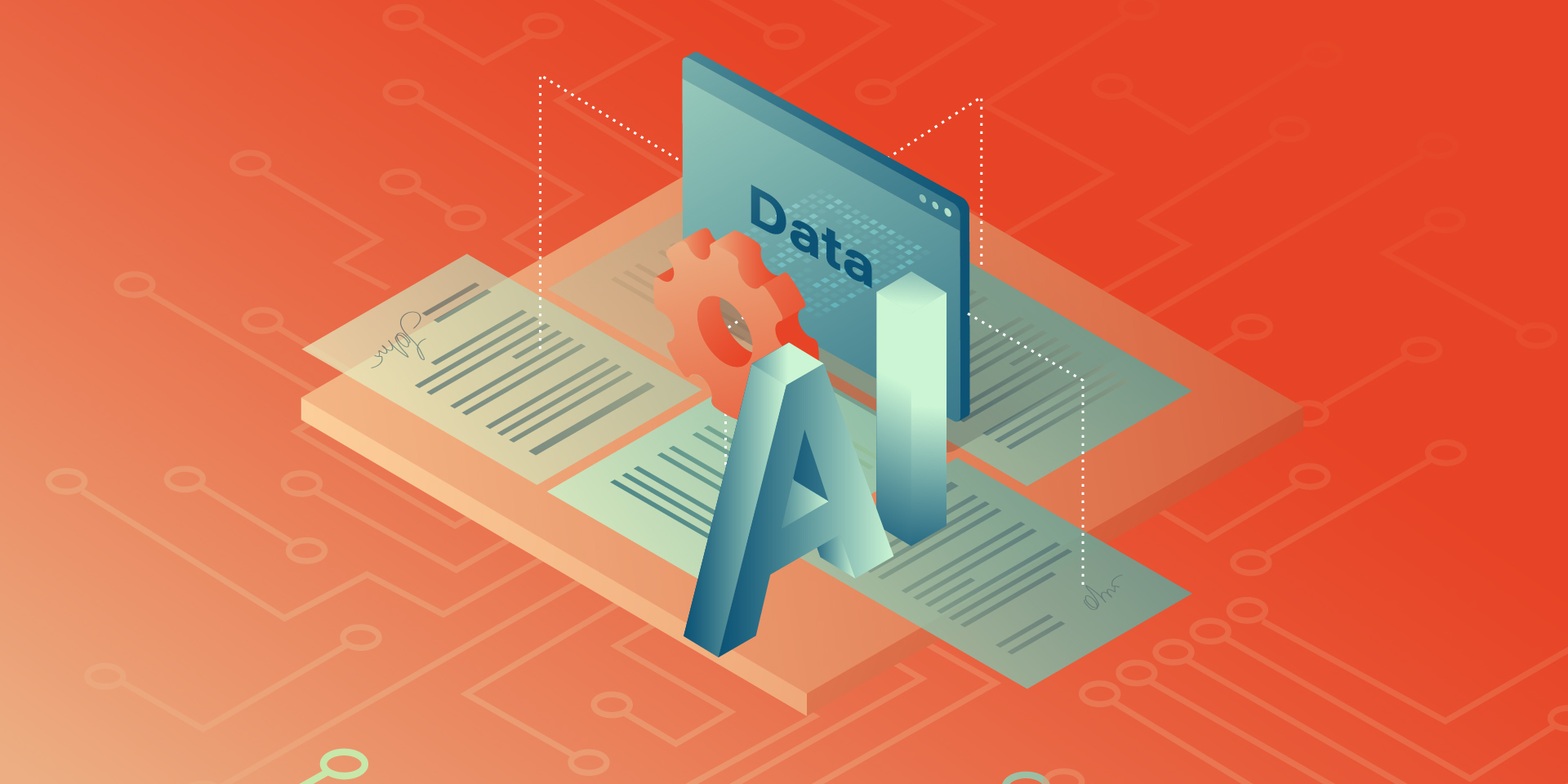
As AI continues to transform industries, one thing becomes increasingly clear: the success of AI-driven initiatives depends not just on algorithms but on the quality and readiness of the data that fuels them. Without well-prepared data, even the most advanced artificial intelligence endeavors can fall short of their promise. In this guide, we cover the practical steps you need to take to prepare your data for AI.
What’s the point of AI-ready data?
The conversation around AI has shifted dramatically in recent years. No longer a distant possibility, AI is now actively changing business landscapes – transforming supply chains through predictive analytics, personalizing customer experiences with advanced recommendation engines, and even assisting in complex fields like financial modeling and healthcare diagnostics.
The focus today is not on whether AI technologies can fulfill its potential but on how organizations can best deploy it to achieve meaningful, scalable business outcomes.
Despite pouring significant resources into AI, businesses are still finding it challenging to fully tap into its economic potential.
For example, according to Gartner, 50% of organizations are actively assessing GenAI’s potential, and 33% are in the piloting stage. Meanwhile, only 9% have fully implemented generative AI applications in production, while 8% do not consider them at all.
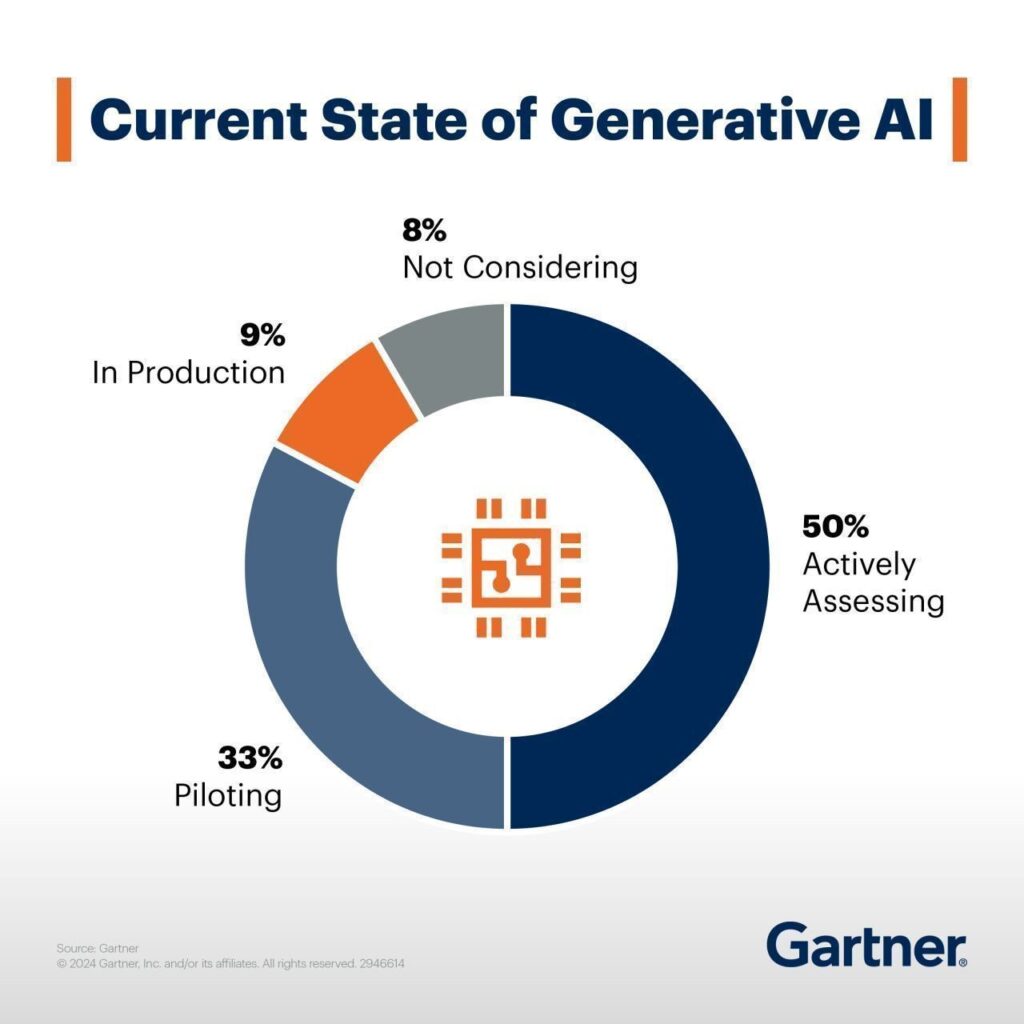
Source: www.gartner.com
The problem often comes down to a key but frequently overlooked factor: the relationship between AI and data. The key issue is the lack of data preparedness. In fact, only 37% of data leaders believe that their organizations have the right data foundation for generative AI, with just 11% agreeing strongly. That means specifically that chief data officers and data leaders need to develop new data strategies and improve data quality to make generative AI work effectively.
What does your business gain by getting your data AI-ready?
When your data is clean, organized, and well-managed, AI can help you make smarter decisions, boost efficiency, and even give you a leg up on the competition.
So, what exactly are the benefits of putting in the effort to prepare your data for AI? Let’s break it down into some real, tangible advantages.
- Clean, organized data allows AI to quickly analyze large amounts of information, helping businesses understand customer preferences, spot market trends, and respond more effectively to changes.
- Getting data AI-ready can save time by automating repetitive tasks and reducing errors.
- When data is properly prepared, AI can offer personalized recommendations and targeted marketing, which can enhance customer satisfaction and build loyalty.
- Companies that prepare their data for AI can move faster, innovate more easily, and adapt better to changes in the market, giving them a clear edge over competitors.
- Proper data preparation ensures businesses can comply with regulations and protect sensitive information.
Importance of data readiness for AI
Unlike traditional algorithms that were bound by predefined rules, modern AI systems learn and adapt dynamically when they have access to data that is both diverse and high-quality.
For many businesses, the challenge is that their data is often trapped in outdated legacy systems that are not built to handle the volume, variety, or velocity required for effective AI. To enable AI to innovate, companies need to first free their data from old silos and establish a proper data infrastructure.
Key considerations for data modernization
- Bring together data from different sources to create a complete picture, which is essential for AI systems to make useful interpretations.
- Build a flexible data infrastructure that can handle increasing amounts of data and adapt to changing AI needs.
- Set up systems to process data in real-time or near-real-time for applications that need immediate insights.
- Consider ethical and privacy issues and comply with regulations like GDPR or CCPA.
- Continuously monitor data quality and AI performance to maintain accuracy and usefulness.
- Employ data augmentation techniques to increase the variety and volume of data for training AI models when needed.
- Create feedback mechanisms to improve data quality and AI performance based on real-world results.
Creating data strategy for AI
Many organizations fall into the trap of trying to apply AI across every function, often ending up with wasted resources and disappointing results. A smarter approach is to start with a focused data strategy.
Think about where AI can truly make a difference – would it be automating repetitive scheduling tasks, personalizing customer experiences with predictive analytics, or using generative AI for content creation and market analysis?
Pinpoint high-impact areas to gain business value without spreading your efforts too thin.
Building a solid AI strategy is also about creating a strong data foundation that brings all factors together. This means making sure your data is not only reliable, secure, and well-organized but also set up to support specific AI use cases effectively.
It also involves creating an environment that encourages experimentation and learning. This way, your organization can continuously adapt, refine its approach, and get the most out of AI over time.
Building an AI-optimized data infrastructure
After establishing an AI strategy, the next step is building a data platform that works like the organization’s central nervous system, connecting all data sources into a unified, dynamic ecosystem.
Why do you need it? Because traditional data architectures were built for simpler times and can’t handle the sheer diversity and volume of today’s data – everything from structured databases to unstructured content like videos, audio, and user-generated data.
An AI-ready data platform needs to accommodate all these different data types while ensuring quick and efficient access so that AI models can work with the most relevant, up-to-date information.
Your data platform needs to show “data lineage” – essentially, a clear map of how data moves through your system. This includes where the data originates, how it’s transformed over time, and how it gets used in the end. Understanding this flow maintains trust in the data, which AI models rely on to make accurate decisions.
At the same time, the platform should support “data liquidity.” This is about breaking data into smaller, manageable pieces that can easily flow between different systems and formats. AI models need this kind of flexibility to get access to the right information when they need it.
Adding active metadata management to this mix provides context, making data easier to interpret and use. When all these components are in place, they turn raw data into a valuable, AI-ready asset.
Setting up data governance and management rules
Think of data governance as defining the rules of the game: how data should be collected, stored, and accessed across your organization. This includes setting up clear policies on data ownership, access controls, and regulatory compliance to protect sensitive information and ensure your data is ethical, unbiased, and trustworthy.
Data management, on the other hand, is all about putting these rules into action. It involves integrating data from different sources, cleaning it up, and storing it securely, all while making sure that high-quality data is always available for your AI projects. Effective data management also means balancing security with access so your team can quickly get to the data they need without compromising privacy or compliance. Together, strong governance and management practices create a fluid, efficient data environment.
The crux of the matter – preparing your data
Remember that data readiness goes beyond just accumulating volume. The key is to make sure that data remains accurate and aligned with the specific AI objectives. Raw data, coming straight from its source, is often filled with errors, inconsistencies, and irrelevant information that can mislead AI models or distort results.
When you handle data with care, you can be confident that your AI systems will deliver tangible business value across the organization.
Focus on the quality of your training data. It needs to be accurate, consistent, and up-to-date. If there are gaps or errors, your AI models will deliver unreliable results. Address these issues by using data cleaning techniques, like filling in missing values (imputation), removing irrelevant information (noise reduction), and ensuring that all entries follow the same format.
Create a solid data foundation that ensures all assets are ready for AI applications. Rising data volumes (think of transaction histories, service requests, or customer records) can quickly overwhelm AI systems if not properly organized. Therefore, make sure your data is well-categorized, labeled, and stored in a format that’s easy for AI to access and analyze.
Also, make a habit of regularly reviewing your data to keep it accurate, relevant, and ready for use.
Preparing data for generative AI
For generative AI, data preparation is even more specialized, as these models require high-quality datasets that are free of errors, diverse and balanced to prevent biased or misleading outputs.
Your dataset should represent a wide range of scenarios, giving the model a thorough base to learn from, which requires incorporating data from multiple sources, demographics, and contexts.
Also, consider that generative AI models often require specific preprocessing steps depending on the type of data and the model architecture. For example, text data might need tokenization, while image data might require normalization or augmentation.
The big picture – get your organization AI-ready too
All your efforts with data and AI tools won’t matter much if your organization isn’t prepared to embrace these changes. The key is building a team that combines tech talent – like data scientists and machine learning experts – with people who understand your business deeply. This means you might need to train and upskill your existing employees to fill gaps.
But there is more – you also need to think about creating a culture that welcomes transformation. Encourage experimentation, cross-team collaboration, and continuous learning. Make sure everyone understands both the potential and the risks of AI. When your team feels confident and aligned with your AI strategy, that’s when you’ll see the real impact of all your hard work.
By focusing on these steps, you create a solid foundation that helps AI deliver real results, whether that’s through better decision-making, improving customer experiences, or staying competitive in a fast-changing market. Preparing your data may take some effort upfront, but it will make a big difference in how well your AI projects perform in the long run.
Check related articles
Read our blog and stay informed about the industry's latest trends and solutions.
see all articles