A Path To a Successful AI Adoption
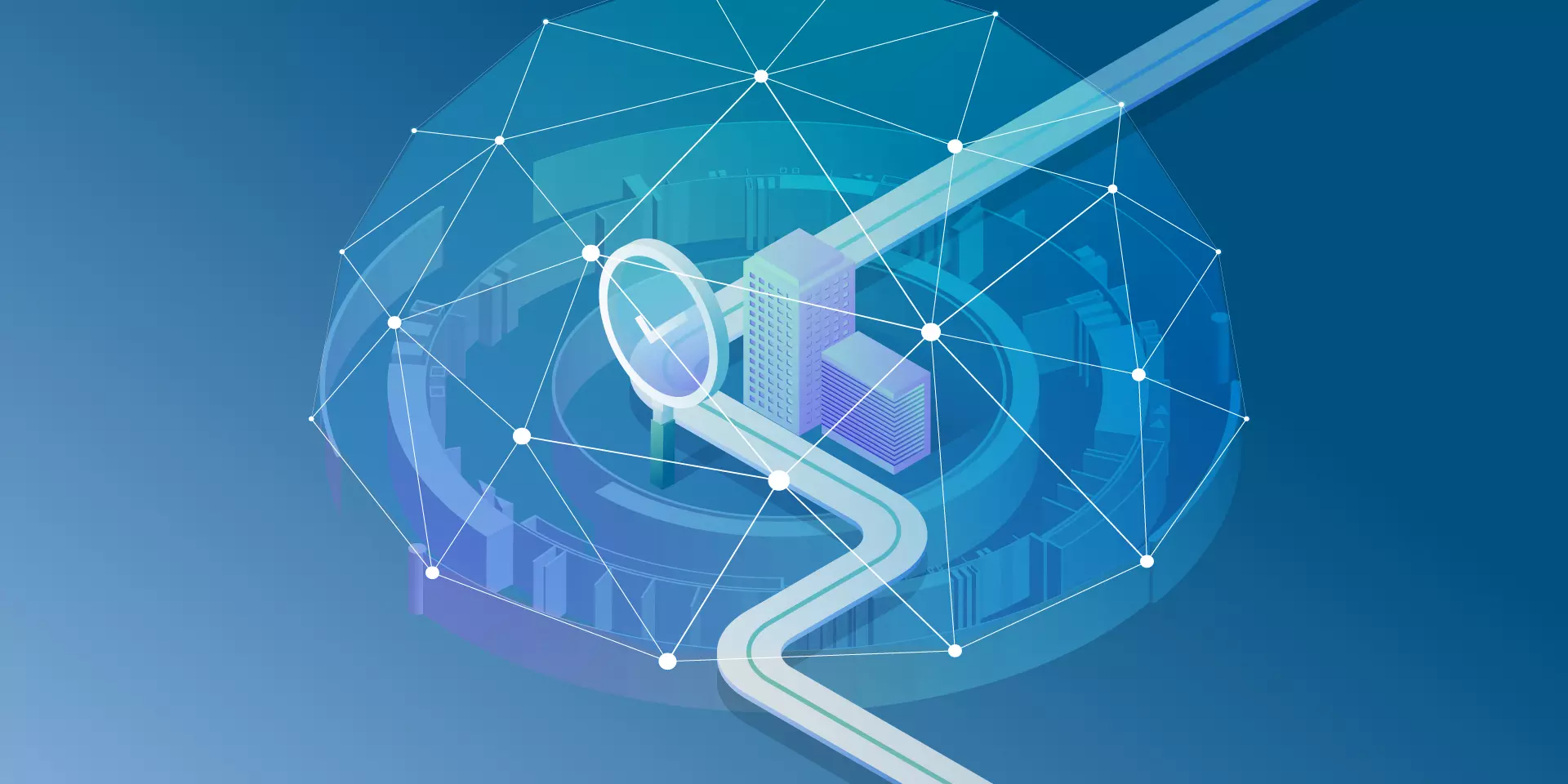
Artificial Intelligence seems to be a quite overused term in recent years, yet it is hard to argue that it is definitely the greatest technological promise of current times. Every industry strongly believes that AI will empower them to introduce innovations and improvements to drive their businesses, increase sales, and reduce costs.
But even though AI is no longer a new thing, companies struggle with adopting and implementing AI-driven applications and systems. That applies not only to large scale implementations (which are still very rare) but often to the very first projects and initiatives within an organization. In this article, we will shed some light on how to successfully adopt AI and benefit from it.
AI adoption – how to start?
How to start then? The answer might sound trivial, but it goes like this: start small and grow incrementally. Just like any other innovation, AI cannot be rolled out throughout the organization at once and then harvested across various business units and departments. The very first step is to start with a pilot AI adoption project in one area, prove its value and then incrementally scale up AI applications to other areas of the organization.
But how to pick the right starting point? A good AI pilot project candidate should have certain characteristics:
- It should create value in one of 3 ways:
- By reducing costs
- By increasing revenue
- By enabling new business opportunities
- It should give a quick win (6-12 months)
- It should be meaningful enough to convince others to follow
- It should be specific to your industry and core business
At Grape Up, we help our customers choose the initial AI project candidate by following a proven process. The process consists of several steps and eventually leads to implementing a single pilot AI project in production.
Step 1: Ideation
We start with identifying possible areas in the organization that might be enhanced with AI, e.g., parts of processes to improve, problems to solve, or tasks to automate. This part of the process is the most essential as it becomes the baseline for all subsequent phases. Therefore it is crucial to execute it together with the customer but also ensure the customer understands what AI can do for their organization. To enable that, we explain the AI landscape, including basic technology, data, and what AI can and cannot do. We also show exemplary AI applications to a customer-specific industry or similar industries.
Having that as a baseline, we move on to the more interactive part of that phase. Together with customer executives and business leaders, we identify major business value drivers as well as current pain points & bottlenecks through collaborative discussion and brainstorming. We try to answer questions such as:
- What in current processes impedes your business development?
- What tasks in current processes are repeatable, manual, and time-consuming?
- What are your pain points, bottlenecks, and inefficiencies in your current processes?
This step results in a list of several (usually 5 to 10) ideas ready for further investigation on where to potentially start applying AI in the organization.
Step 2: Business Value Evaluation
The next step aims at detailing the previously selected ideas. Again, together with the customer, we define detailed business cases describing how problems identified in step 1 could be solved and how these solutions can create business value.
Every idea is broken down into a more detailed description using the Opportunity Canvas approach – a simple model that helps define the idea better and consider its business value. Using filled canvas as the baseline, we analyze each concept and evaluate against the business impact it might deliver, focusing on business benefits and user value but also expected effort and cost.
Eventually, we choose 4-8 ideas with the highest impact and the lowest effort and describe detailed use cases (from business and high-level functional perspective).
Step 3: Technical evaluation
In this phase, we evaluate the technical feasibility of previously identified business cases – in particular, whether AI can address the problem, what data is needed, whether the data is available, what is the expected cost and timeframe, etc.
This step usually requires technical research to identify AI tools, methods, and algorithms that could best address the given computational problem, data analysis – to verify what data is needed vs. what data is available and often small-scale experiments to better validate the feasibility of concepts.
We finalize this phase with a list of 1-3 PoC candidates that are technically feasible to implement but more importantly – are verified to have a business impact and to create business value.
Step 4: Proof of Concept
Implementation of the PoC project is the goal of this phase and involves data preparation (to create data sets whose relationship to the model targets is understood), modeling (to design, train, and evaluate machine learning models), and eventual deployment of the PoC model that best addresses the defined problem.
It results in a working PoC that creates business value and is the foundation for the production-ready implementation.
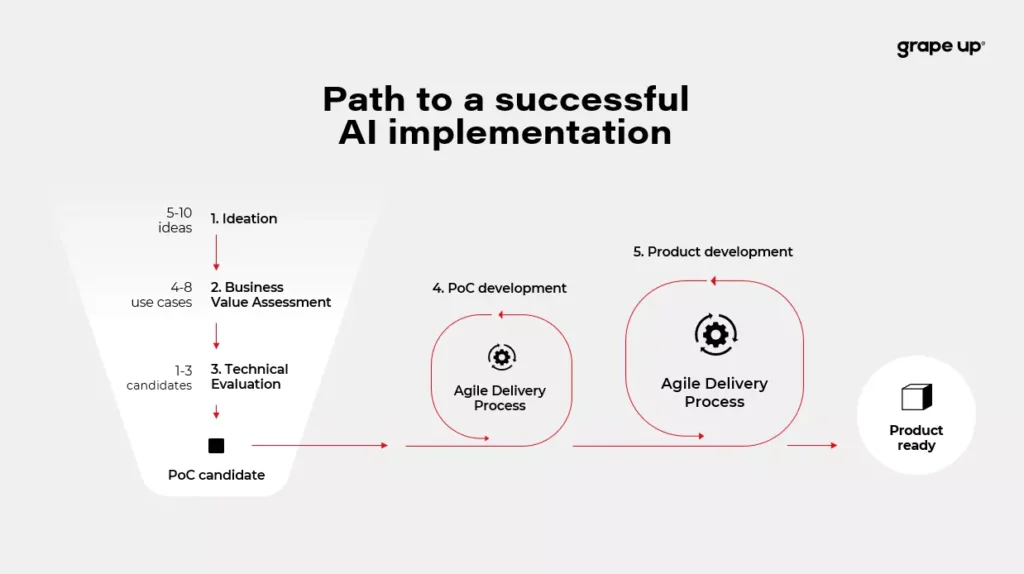
How to move AI adoption forward?
Once the customer is satisfied with PoC results, they want to productionize the solution to fully benefit from the AI-driven tool. Moving pilots to production is also a crucial part of scaling up AI adoption. If the successful projects remain still just experiments and PoCs, then it is demanding for a company to move forward and apply AI to other processes within the organization.
To summarize the most important aspects of a successful AI adoption:
- Start small – do not try to roll out innovation globally at once.
- Begin with a pilot project – pick the meaningful starting point that provides business value but also is feasible.
- Set realistic expectations – do not perceive AI as the ultimate solution for all your problems.
- Focus on quick wins – choose a solution that can be built within 6-12 months to quickly see the results and benefits.
- Productionize – move out from the PoC phase to production to increase visibility and business impact.
Check related articles
Read our blog and stay informed about the industry's latest trends and solutions.
see all articles
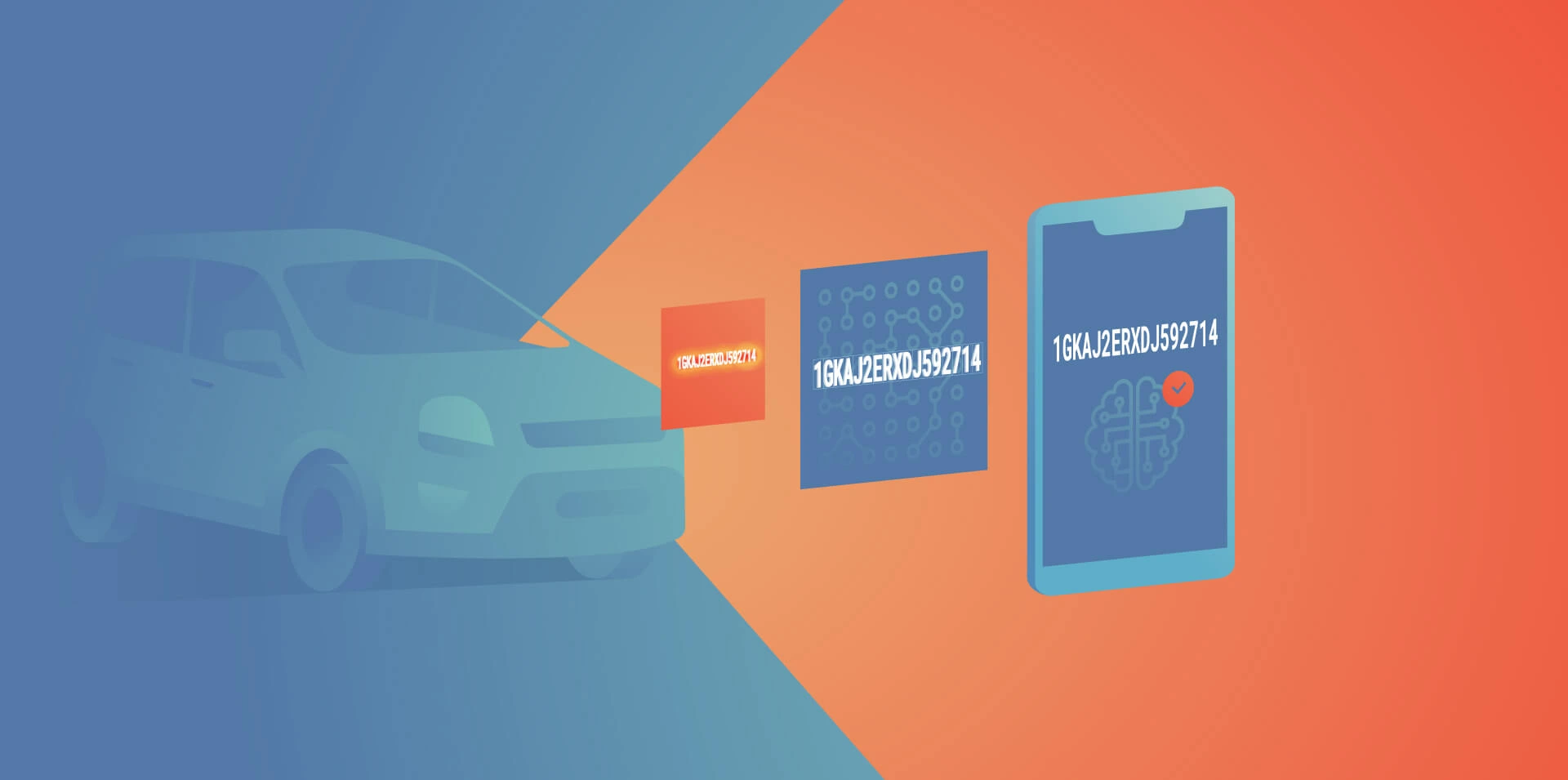
Leveraging AI To Improve VIN Recognition – How To Accelerate and Automate Operations in the Insurance Industry
Read the article