The Key to ROAI: Why High-Quality Data Is the Real Engine of AI Success
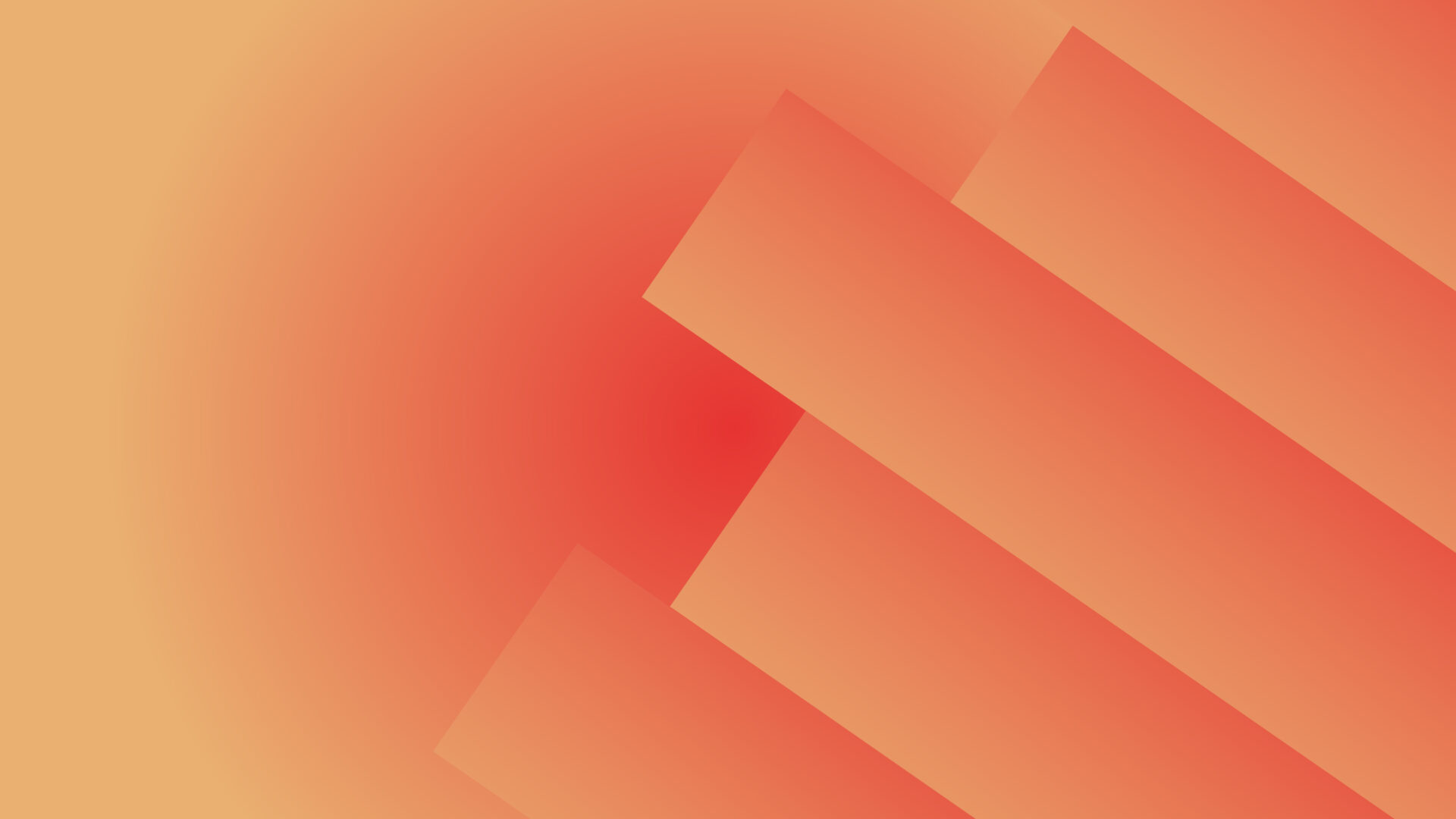
Data might not literally be “the new oil,” but it’s hard to ignore its growing impact on companies’ operations. By some estimates, the world will generate over 180 zettabytes of data by the end of 2025. Yet, many organizations still struggle to turn that massive volume into meaningful insights for their AI projects.
According to IBM, poor data quality already costs the US economy alone $3.1 trillion per year – a staggering figure that underscores just how critical proper governance is for any initiative, AI included.
On the flip side, well-prepared data can dramatically boost the accuracy of AI models, shorten the time it takes to get results and reduce compliance risks. That’s why the high quality of information is increasingly recognized as the biggest factor in an AI project’s success or failure and a key to ROAI.
In this article, we’ll explore why good data practices are so vital for AI performance, what common pitfalls often derail organizations, and how usage transparency can earn customer trust while delivering a real return on AI investment.
Why data quality dictates AI outcomes
An AI model’s accuracy and reliability depend on the breadth, depth, and cleanliness of the data it’s trained on. If critical information is missing, duplicated, or riddled with errors, the model won’t deliver meaningful results, no matter how advanced it is. It’s increasingly being recognized that poor quality leads to inaccurate predictions, inefficiencies, and lost opportunities.
For example, when records contain missing values or inconsistencies, AI models generate results that don’t reflect reality. This affects everything from customer recommendations to fraud detection, making AI unreliable in real-world applications. Additionally, poor documentation makes it harder to trace data sources, increasing compliance risks and reducing trust in AI-driven decisions.
The growing awareness has made data governance a top priority across industries as businesses recognize its direct impact on AI performance and long-term value.
Metrics for success: Tracking the impact of quality data on AI
Even with the right data preparation processes in place, organizations benefit most when they track clear metrics that tie data quality to AI performance. Here are key indicators to consider:
Monitoring these metrics lets organizations gain visibility into how effectively their information supports AI outcomes. The bottom line is that quality data should lead to measurable gains in operational efficiency, predictive accuracy, and overall business value. In other words – it’s the key to ROAI.
However, even with strong data quality controls, many companies struggle with deeper structural issues that impact AI effectiveness.
AI works best with well-prepared data infrastructures
Even the cleanest sets won’t produce value if data infrastructure issues slow down AI workflows. Without a strong data foundation, teams spend more time fixing errors than training AI models.
Let’s first talk about the people – they too are, after all, key to ROAI.
The right talent makes all the difference
Fixing data challenges is about tools as much as it is about people.
- Data engineers make sure AI models work with structured, reliable datasets.
- Data scientists refine data quality, improve model accuracy, and reduce bias.
- AI ethicists help organizations build responsible, fair AI systems.
Companies that invest in data expertise can prevent costly mistakes and instead focus on increasing ROAI.
However, even with the right people, AI development still faces a major roadblock: disorganized, unstructured data.
Disorganized data slows AI development
Businesses generate massive amounts of data from IoT devices, customer interactions, and internal systems. Without proper classification and structure, valuable information gets buried in raw, unprocessed formats. This forces data teams to spend more time cleaning and organizing instead of implementing AI in their operations.
- How to improve it: Standardized pipelines automatically format, sort, and clean data before it reaches AI systems. A well-maintained data catalog makes information easier to locate and use, speeding up development.
Older systems struggle with AI workloads
Many legacy systems were not built to process the volume and complexity of modern AI workloads. Slow query speeds, storage limitations, and a lack of integration with AI tools create bottlenecks. These issues make it harder to scale AI projects and get insights when they are needed.
- How to improve it: Upgrading to scalable cloud storage and high-performance computing helps AI process data faster. Moreover, integrating AI-friendly databases improves retrieval speeds and ensures models have access to structured, high-quality inputs.
Beyond upgrading to cloud solutions, businesses are exploring new ways to process and use information.
- Edge computing moves data processing closer to where it’s generated to reduce the need to send large volumes of information to centralized systems. This is critical in IoT applications, real-time analytics, and AI models that require fast decision-making.
- Federated learning allows AI models to train across decentralized datasets without sharing raw data between locations. This improves security and is particularly valuable in regulated industries like healthcare and finance, where data privacy is a priority.
Siloed data limits AI accuracy
Even when companies maintain high-quality data, access restrictions, and fragmented storage prevent teams from using it effectively. AI models trained on incomplete datasets miss essential context, which in turn leads to biased or inaccurate predictions. When different departments store data in separate formats or systems, AI cannot generate a full picture of the business.
- How to improve it: Breaking down data silos allows AI to learn from complete datasets. Role-based access controls provide teams with the right level of data availability without compromising security or compliance.
Fixing fragmented data systems and modernizing infrastructure is key to ROAI, but technical improvements alone aren’t enough. Trust, compliance, and transparency play just as critical a role in making AI both effective and sustainable.
Transparency, privacy, and security: The trust trifecta
AI relies on responsible data handling. Transparency builds trust and improves outcomes, while privacy and security keep organizations compliant and protect both customers and businesses from unnecessary risks. When these three elements align, people are more willing to share data, AI models become more effective, and companies gain an edge.
Why transparency matters
82% of consumers report being “highly concerned” about how companies collect and use their data, with 57% worrying about data being used beyond its intended purpose. When customers understand what information is collected and why, they’re more comfortable sharing it. This leads to richer datasets, more accurate AI models, and smarter decisions. Internally, transparency helps teams collaborate more effectively by clarifying data sources and reducing duplication.
Privacy and security from the start – a key to ROAI
While transparency is about openness, privacy and security focus on protecting data. Main practices include:
Compliance as a competitive advantage
Clear records and responsible data practices reduce legal risks and allow teams to focus on innovation instead of compliance issues. Customers who feel their privacy is respected are more willing to engage, while strong data practices can also attract partners, investors, and new business opportunities.
Use data as the strategic foundation for AI
The real value of AI comes from turning data into real insights and innovation – but none of that happens without a solid data foundation.
Outdated systems, fragmented records, and governance gaps hold back AI performance. Fixing these issues ensures AI models are faster, smarter, and more reliable.
Are your AI models struggling with data bottlenecks?
Do you need to modernize your data infrastructure to support AI at scale?
We specialize in building, integrating, and optimizing data architectures for AI-driven businesses.
Let’s discuss what’s holding your AI back and how to fix it.
Contact us to explore solutions tailored to your needs.
Check related articles
Read our blog and stay informed about the industry's latest trends and solutions.
see all articles