The Foundation for AI Success: How to Build a Strategy to Increase ROAI
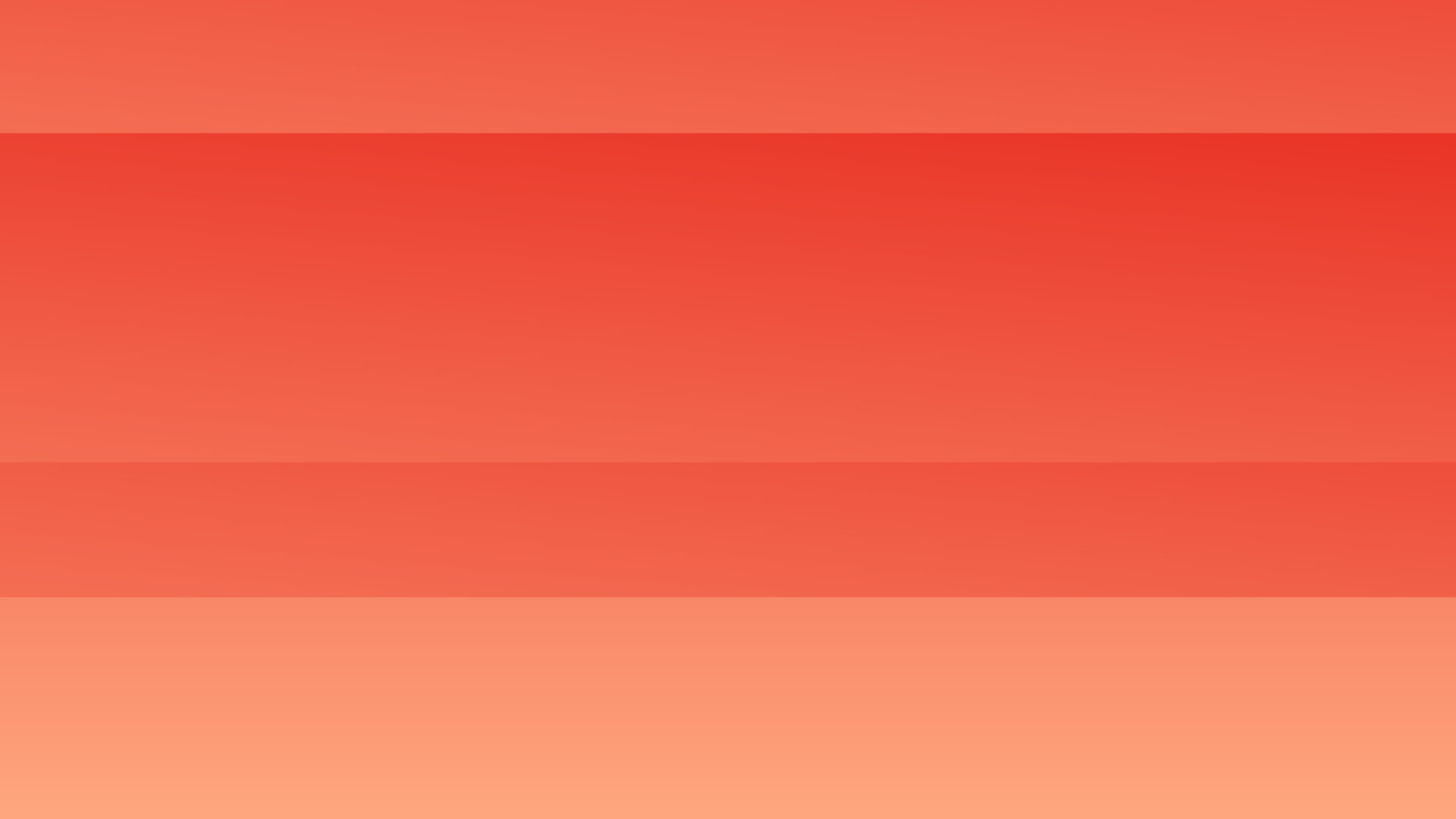
AI adoption is on the rise but turning it into real business value is another story. 74% of companies struggle to scale AI initiatives, and only a tiny fraction – just 26% – develop the capabilities needed to move beyond proofs of concept. The real question on everyone’s mind is – How to increase ROAI?
One of the biggest hurdles is proving the impact. In 2023, the biggest challenge for businesses was demonstrating AI’s usefulness in real operations. Many companies invest in this technology without a clear plan for how it will drive measurable results.
Even with these challenges, the adoption keeps growing. McKinsey’s 2024 Global Survey on AI reported that 65% of respondents’ organizations are regularly using Generative AI in at least one business function, nearly doubling from 33% in 2023. Businesses know its value, but making artificial intelligence work at scale takes more than just enthusiasm.
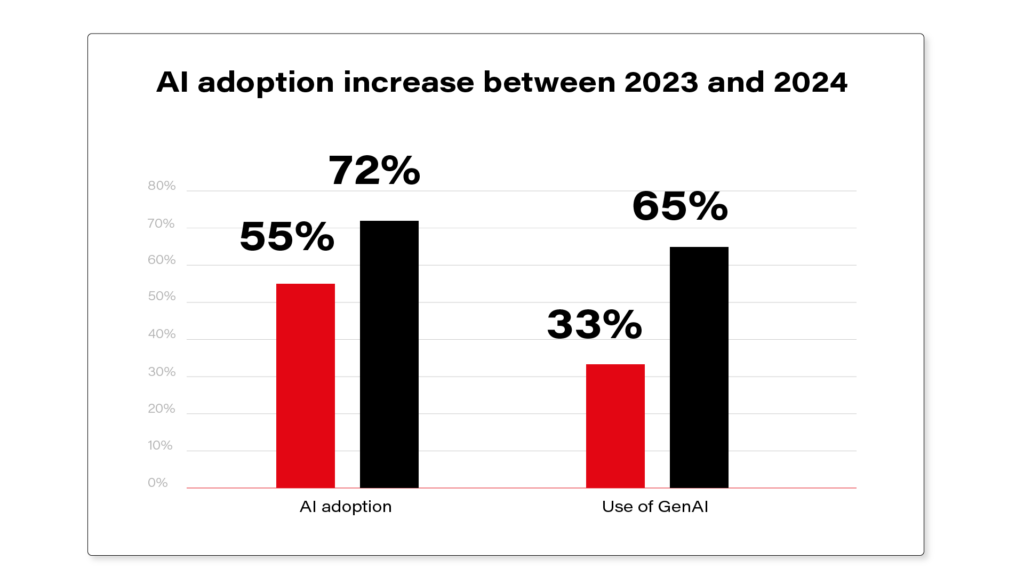
Source: https://www.mckinsey.com/capabilities/quantumblack/our-insights/the-state-of-ai
That’s where the right approach makes all the difference. A holistic strategy, strong data infrastructure, and efficient use of talent can help you increase ROAI and turn technology into a competitive advantage. But you need to start with building a foundation for AI investments and implementation first.
Why AI must be aligned with business goals
Too many AI projects fail when companies focus on the technology first instead of the problem it’s meant to solve. Investing in artificial intelligence just because it’s popular leads to expensive pilots that never scale, systems that complicate workflows instead of improving them, and wasted budgets with nothing to show for it.
Start with the problem, not the technology
Before committing resources, leadership needs to ask:
- What’s the goal? Is the priority cutting maintenance costs, making faster decisions, or detecting fraud more accurately? If the objective isn’t clear, neither will the results.
- Is AI even the right solution? Some problems don’t need machine learning. Sometimes, better data management or process improvements do the job just as well, without the complexity or cost of AI.
Choosing AI use cases that deliver real value
Once AI aligns with business goals, the next challenge is selecting initiatives that generate measurable impact. Companies often waste millions on projects that fail to solve real business problems, can’t scale, or disrupt workflows instead of improving them.
See which factors must align for AI to create tangible business value:
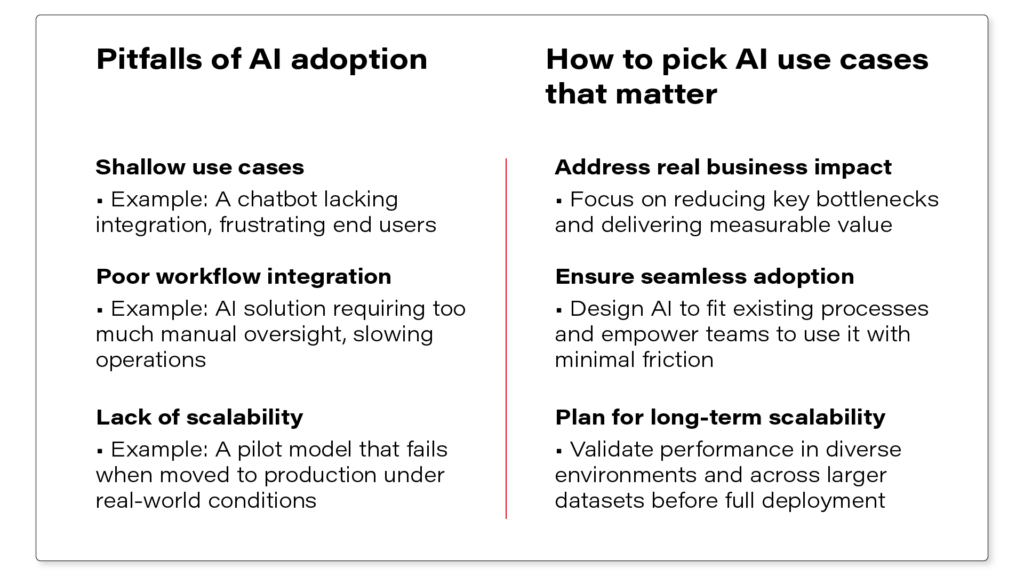
How responsible AI ties back to business results
Responsible AI protects long-term business value by creating systems that are transparent, fair, and aligned with user expectations and regulatory requirements. Organizations that take a proactive approach to AI governance minimize risks while building solutions that are both effective and trusted.
One of the biggest gaps in AI adoption is the lack of consistent oversight. Without regular audits and monitoring, models can drift, introduce bias, or generate unreliable results. Businesses need structured frameworks to keep AI reliable, adaptable, and aligned with real-world conditions. This also means actively managing ethical issues, explainability, and data security to maintain performance and trust.
As regulations evolve, compliance is no longer an afterthought. AI used in critical areas like fraud detection, risk assessment, and automated decision-making requires continuous monitoring to meet regulatory expectations. Companies that embed AI governance from the start avoid operational risks.
Another key challenge is trust. When AI-driven decisions lack transparency, scepticism grows. Users and stakeholders need clear visibility into how AI operates to build confidence. Companies that make decisions transparent and easy to understand improve adoption across their organization, and ultimately increase ROAI.
Measuring AI success and proving ROAI
The real test of AI’s success is whether it improves daily operations and delivers measurable business value. When teams work more efficiently, revenue grows, and risks become easier to manage, the investment is clearly paying off.
Key indicators of AI success
Is AI reducing manual effort? Automating repetitive tasks helps employees focus on more strategic work. If delays still slow operations or fraud detection overwhelms teams with false positives, AI may not be delivering real efficiency. Faster approvals and quicker customer issue resolution indicate AI is making a difference.
Is AI improving financial outcomes? Accurate forecasting cuts waste, and AI-driven pricing boosts profit margins. If automation isn’t lowering operational costs or streamlining workflows, it may not be adding real value.
Is AI strengthening security and compliance? Fraud detection prevents financial losses when it catches real threats without unnecessary disruptions. Compliance automation eases the burden of manual oversight, while AI-driven security reduces the risk of data breaches. If risks remain high, AI may need adjustments.
To prove AI’s return on investment, companies need to establish success criteria upfront, track AI performance over time, and compare different configurations (e.g., Generative AI use cases, LLM models) to confirm the technology delivers cost savings and tangible benefits.
The hidden costs of AI initiatives and the challenge of scaling
Investing in artificial intelligence goes beyond development. Many companies focus on building and implementing models but underestimate the effort required to scale, maintain, and integrate them into existing systems. Costs accumulate over time, and without proper planning, AI projects can stall, and budgets stretch.
One of the highest ongoing costs is data. AI relies on clean, structured information, but collecting, storing, and maintaining it requires continuous effort. Over time, models need regular updates to remain accurate as well. Fraud tactics change, regulations evolve, and systems produce unreliable results without adjustments, leading to costly mistakes.
This becomes even more challenging when AI moves from a controlled pilot to full-scale implementation. A model that performs well in one department may not integrate easily across an entire organization. Expanding its use often exposes hidden costs, workflow disruptions, and technical limitations that weren’t an issue on a smaller scale.
Scaling AI successfully also requires coordination across different teams. While ML engineers refine models, business teams track measurable outcomes, and compliance teams manage regulatory requirements. You need these groups to align early.
AI must also integrate with existing enterprise systems without disrupting workflows, which requires dedicated infrastructure investments. Many legacy IT environments weren’t designed for AI-driven automation, which leads to increased costs for adaptation, cloud migration, and security improvements.
Companies that navigate these challenges effectively see real gains from AI. However, aligning strategy, execution, and scaling AI efficiently isn’t always straightforward. That’s where expert guidance makes a difference.
See how Grape Up helps businesses increase ROAI
Grape Up helps business leaders turn AI from a concept into a practical tool that delivers measurable ROAI by aligning technology with real business needs.
We work with companies to define AI roadmaps, making sure every initiative has a clear purpose and contributes to strategic goals. Our team supports data infrastructure and AI integration, so new solutions fit smoothly into existing systems without adding complexity.
From strategy to execution, Grape Up helps you increase ROAI. Make technology a real business asset adapted for long-term success.
Check related articles
Read our blog and stay informed about the industry's latest trends and solutions.
see all articles