How Predictive Maintenance Changes the Automotive Industry
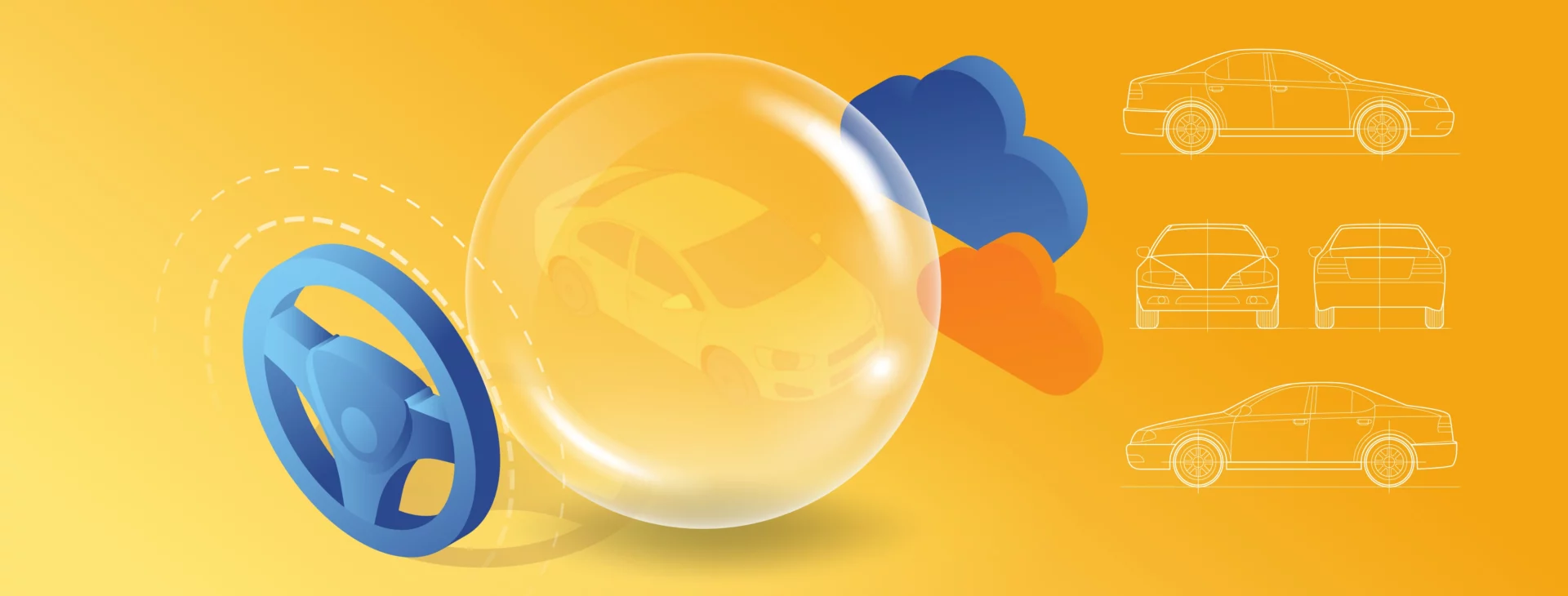
Ever since Henry Ford implemented the first production line and launched mass production of the Ford Model T, the automotive industry has been on the constant lookout for ways to boost performance. This aspect has become even more relevant today, given the constant market and social unrest. Coming to rescue supply chain management and product lifecycle optimization is predictive maintenance. Not only OEMs, but the entire automotive industry: insurers, car rental companies and vehicle owners are benefiting from the implementation of this technology.
Predictive Maintenance Explained
Predictive maintenance is an advanced maintenance approach that utilizes data science and predictive analytics to anticipate when equipment or machinery requires maintenance before it faces a breakdown.
The primary aim is to schedule maintenance at optimal times, considering convenience and cost-effectiveness while maximizing the equipment’s longevity. By identifying potential issues before they become critical, predictive maintenance significantly reduces the likelihood of equipment breakdowns.
Various types of maintenance strategies are employed in different industries:
- Reactive Maintenance: Also known as “run-to-failure,” this method involves waiting for equipment to fail before conducting maintenance. Therefore, unscheduled downtime and higher repair costs may occur.
- Periodic Maintenance: This approach entails performing maintenance tasks at regular intervals, regardless of the equipment’s condition. It helps prevent unexpected breakdowns but may lead to unnecessary maintenance if done too frequently.
- Smart Maintenance: Smart maintenance utilizes advanced technologies like IoT devices and sensors to monitor equipment in real-time and identify anomalies or potential failures.
- Condition-Based Maintenance: This strategy relies on monitoring the equipment’s condition while it is in operation. Maintenance is only carried out when data indicates a decline in performance or a deviation from normal parameters, optimizing maintenance schedules and reducing unnecessary work.
- Predictive Maintenance: The most advanced type of maintenance uses real-time operational data and predictive analytics to forecast when maintenance is required. It aims to schedule maintenance before equipment failure occurs based on data-driven predictions, thus minimizing downtime, reducing costs, and prolonging equipment lifespan.
Predictive maintenance employs various techniques, such as vibration analysis, acoustic monitoring, infrared technology, oil analysis, and motor circuit analysis. These methods enable continuous equipment condition monitoring and early detection of potential failures, facilitating timely maintenance interventions.
Differentiation between predictive maintenance and preventive maintenance
Predictive maintenance hinges on the real-time condition of assets and is implemented only when the need arises. Its purpose is to anticipate potential failures by monitoring assets while they are actively operational. Unlike preventive maintenance, this approach is rooted in the current operational state of an asset rather than statistical analysis and predetermined schedules.
Essential steps in creating a predictive maintenance solution
Predictive maintenance solutions utilize a combination of sensors, artificial intelligence, and data science to optimize equipment maintenance.
The development of such solutions varies depending on equipment, environment, process, and organization, leading to diverse perspectives and technologies guiding their creation. However, there are steps common to every project: data collection and analysis, model development and deployment, as well as continuous improvement.
Here is a step-by-step process of how solutions are developed in the automotive industry:
- Data Collection: Relevant data is collected from sensors, equipment logs, vehicle diagnostics, telemetry, and other sources. This data includes information about the performance, condition, and behavior of the vehicles, such as engine temperature, fuel consumption, mileage, and more. Telematics systems can provide real-time data on vehicle location, speed, and usage patterns, while maintenance logs record historical maintenance activities, repairs, and part replacements.
- Data Preprocessing: The collected data is organized, and prepared for analysis. Data preprocessing involves cleaning the data by removing outliers or erroneous values, handling missing values through imputation or interpolation, and converting the data into a suitable format for analysis.
- Feature Engineering: Important features or variables that can provide insights into the health and performance of the vehicles are selected from the collected data. These features can include engine vibration, temperature, fuel consumption, mileage, and more. Feature selection step involves identifying the most relevant features that have a strong correlation with the target variable (e.g., equipment failure). It helps to reduce the dimensionality of the data and improve the model’s efficiency and interpretability. Later, selected features are transformed to make them more suitable for modelling. The process may include techniques such as logarithmic or exponential transformations, scaling, or encoding categorical variables.
- Model Development: Machine learning algorithms are applied to the selected features to develop predictive models. These models learn from historical data and identify patterns and relationships between various factors and equipment failures. The algorithms used can include regression, decision trees, random forests, neural networks, and more.
- Model Training and Validation: The developed models are trained using historical data and validated to ensure their accuracy and performance. This involves splitting the data into training and testing sets, evaluating the model’s performance metrics, and fine-tuning the model if necessary.
- Deployment and Monitoring: The trained models are deployed into the predictive maintenance system, which continuously monitors real-time data from sensors and other sources. Telematics systems are used to collect GPS and vehicle-specific data, which it transmits through different methods (cellular network, satellite communication, 4G mobile data, GPRS) to the central server. The system detects anomalies, recognizes patterns, and provides insights into the health of the vehicles. It can alert maintenance teams when potential issues are detected.
- Continuous Improvement: The predictive maintenance solution is continuously improved by collecting feedback, monitoring its performance, and updating the models and algorithms as new data becomes available.
Most common problems in deploying predictive maintenance solutions
Implementing predictive maintenance solutions in a fleet of vehicles or in a vehicle factory is a process that requires time, consistency and prior testing. Among the main challenges of rolling out this technology, the following aspects in particular are noteworthy.
Data integration
Integrating data from many sources is a significant barrier to implementing predictive maintenance solutions. To accomplish this with a minimum delay and maximum security, it is necessary to streamline the transfer of data from machines to ERP systems. To collect, store, and analyze data from many sources, businesses must have the proper infrastructure in place.
Insufficient data
Lack of data is a major hindrance to implementing predictive maintenance systems. Large amounts of information are needed to develop reliable models for predictive maintenance. Inadequate information might result in inaccurate models, which in turn can cause costly consequences like premature equipment breakdowns or maintenance.
To get over this difficulty, businesses should collect plenty of data for use in developing reliable models. They should also check that the data is relevant to the monitored machinery and of high quality. Businesses can utilize digital twins, or digital representations of physical assets, to mimic the operation of machinery and collect data for use in predictive maintenance systems.
Process complexity
Transitioning from preventive to predictive maintenance is complex and time-intensive. It requires comprehensive steps beyond technology, including assembling a skilled team and managing upfront costs. Without qualified experts versed in software and process intricacies, project success is doubtful.
High costs
The implementation of predictive maintenance programs comes with substantial costs. These upfront expenses pose challenges, including the need to invest in specialized sensors for data collection, procure effective data analysis tools capable of managing complexity, and possibly hire or train personnel with technical expertise.
To address these hurdles, collaboration with specialized vendors and the utilization of cloud-based solutions can prove cost-effective. Additionally, digital twin technology offers a way to simulate equipment behavior and minimize reliance on physical sensors, potentially reducing overall expenses.
Privacy and security issues
The implementation of predictive maintenance involves extensive data collection and analysis, which can give rise to privacy concerns. Companies must adhere to applicable data protection laws and regulations, and establish proper protocols to safeguard the privacy of both customers and employees. Even though predictive maintenance data may be anonymized and not directly linked to specific individuals, it still necessitates robust security measures, since preventing data breaches and unauthorized access to vital company information is crucial for overall success.
What Are the Benefits of Predictive Maintenance?
Life cycle optimization, stock management, or even recycling management – in each of these fields predictive maintenance can bring substantial benefits. And this is not only for OEMs but also for fleet operators, transportation or logistics companies. And even for the end user.
Below we list the key benefits of implementing predictive maintenance in an automotive-related company:
- Extended lifespan: Predictive maintenance technology detects early signs of wear and potential malfunctions in-vehicle components such as engines, transmissions, and brakes. By addressing these issues proactively, vehicles experience fewer major breakdowns and continue to operate efficiently over a longer period.
- Cost savings: By addressing issues at an early stage, automotive companies can avoid expensive breakdowns and prevent further damage. This proactive approach not only reduces the need for costly replacement parts but also minimizes the labor and operational costs associated with major repairs, resulting in significant long-term cost savings.
- Minimized downtime: Through continuous monitoring and analysis, predictive maintenance predicts when maintenance or repairs are needed and schedules them during planned downtime. This minimizes the likelihood of unexpected breakdowns that can disrupt operations and lead to extended periods of vehicle inactivity. By strategically timing maintenance activities, vehicles spend more time on the road.
- Increased efficiency: Any iissues are detected early, enabling timely corrective actions. This proactive approach leads to improved fuel economy, reduced emissions, and overall enhanced efficiency. Vehicles operate at their peak performance, contributing to a more sustainable and environmentally friendly fleet.
- Enhanced security: Constant monitoring for abnormal vibrations, temperature variations, and fluid leaks ensures that potential issues compromising vehicle safety and security are detected promptly. By addressing these concerns before they escalate, predictive maintenance contributes to ensuring the security of both the vehicle and its occupants. This feature is particularly valuable in critical applications where reliable vehicle performance is paramount, such as emergency response scenarios.
- Avoiding over-maintenance: If you over-maintain corporate resources, it can have the same negative consequences as when failing to maintain them on time. With predictive maintenance, you can focus on maintaining crucial resources at the best possible time and with the best possible results.
- Compliance with required standards and regulations: Laws and regulations related to vehicle production are constantly evolving and pushing OEMs to make numerous production changes (e.g. the legislation related to EV production). Predictive maintenance allows you to better suit the new expectations of legislators and monitor the points of production that are most dependent on the legal context.
- Easier management of parts and materials: As connected cars diagnostic systems become more sophisticated, drivers have the option to make small repairs sooner and keep their vehicles in a better condition. All this means that OEMs and licensed repair shops need fewer parts and can better manage supply chains.
Predictive maintenance clearly is not a one-size-fits-all solution for all sectors. Notably, it will work well for high production volumes and short lead times and anywhere you need to ensure reliability, security and convenience.
The automotive industry is a perfect fit for this model. As shown in the examples featured in the second part of the article, the top players in the market are tapping into this technology.
According to Techsci Research, “The global predictive maintenance market was valued at USD 4.270 billion in 2020 and is projected to grow around USD 22.429 billion by 2026”.
Check related articles
Read our blog and stay informed about the industry's latest trends and solutions.
see all articles